AI is disrupting conventional investment processes: investors should reassess existing as well as new investments
New AI-enabled capabilities affect the whole TMT ecosystem
The TMT industry has always sought to combine its own digital prowess and data resources to provide customer insights and boost operational efficiency. The rise of GenAI has renewed this focus, while also creating a surge in demand for the high-performance computing (HPC) services it relies on, as well as paving the way for the introduction of a new range of consumer services and increasing the personalisation of existing services.
Early use cases indicate GenAI may at last deliver on the promise of cost savings enabled by AI not only in internal operations but also in customer-facing functions, including sales and customer service.1 Nevertheless, reports of inaccuracies in responses and even legal challenges underscore the technology's evolving nature.2,3
Future advances in GenAI will expand beyond text, integrating with voice commands, personalised video content, and interactive gaming to enable novel user experiences. Demand for reduced latency and lower operational costs for these media-rich, interactive services will reshape the demand for HPC services. Demand looks poised to grow for inferencing-focused hardware (with lower energy consumption) and edge data centres (to improve latency).
For many, existing product lines will be also affected, as AI expansion fuels competition in the form of product differentiation and personalisation. Some integral areas of the user experience, such as customer service, will be completely transformed; others will be repackaged, for example by combining legacy products into new bundles with AI-enabled services.
Figure 1: GenAI’s impact on the TMT sector
Some business and operational models will gain more than others
AI’s impact will not be uniform: for example, media companies are already generating tangible benefits from GenAI in the early stages of content production, and this will intensify as consumers adopt new forms of interactive and personalised entertainment.
Functions with significant customer interaction (such as marketing, sales and customer operations) also stand to experience much greater benefits than other areas of the business. A wholesale fibre network operator, for example, may see a smaller impact than a service provider that engages directly with retail consumers.
AI developments generate risk as well as reward
Given the breadth of AI’s impact across the TMT ecosystem, the associated risks are also extensive. They encompass strategic, ethical, regulatory and technical aspects that may directly affect company valuation.
Strategic risks revolve around AI-induced market changes. A shift towards AI-enhanced services may decrease the appeal of traditional products, and companies using AI to reduce operational costs could intensify price competition. Operational efficiencies enabled by AI may reshape staff requirements, and associated job displacement may present ethical challenges related to employee and public relations.
Greater regulatory scrutiny of AI increases risks around non-compliance, and liability for incidents triggered by AI. Examples include intellectual property risks and risk of loss of confidentiality if data used by AI systems can ‘leak’. Beyond data security, technical risks include insufficient safety measures and quality control as new areas of operations are automated, including customer interactions.
Maturity makes a big difference
Most industry participants agree GenAI will have a significant impact on the TMT sector, but adoption currently varies significantly.
Size matters: larger players, traditionally more involved with internal processes and automation, have been quicker than their smaller counterparts to adopt AI;6 however, size brings complexity which may make their journey to reap the benefits of AI a longer one.
Maturity is also a factor: early use cases target cost reduction and operational efficiency, but subsequently give way to more transformational capabilities that allow innovation in business models and the unlocking of new revenue streams. Over time, therefore, AI applications are likely to shift from cost saving to value creation.
Investors need to change the way they review assets
Macroeconomic uncertainty and high interest rates reduced the TMT deal flow in recent years, but there are now signs of returning growth. In 2024’s market environment, there is a clear imperative for buyers and sellers to thoroughly review the implications of recent advances in AI for new transactions. Given the extent of AI’s disruptive potential, it would also be wise to conduct a review of existing assets, to allow early identification of any necessary course correction, and ensure optimal outcomes when these assets are brought to market.
- Market reviews should be performed to assess AI-driven demand over the short term (as the industry applies AI to internal processes and customer interactions) and the long term (as AI becomes an integral part of product portfolios). Competition should be considered for existing players that may use AI to reduce operational costs and compete on the basis of price or by new features, as well as new entrants using AI to unbundle traditional market offerings.
- Business and operational model reviews should assess AI impact in line with market context (e.g. consumers favouring digital touch points), business model (e.g. wholesale versus retail) and operating model (e.g. outsourcing of customer service). Benchmarking should include comparison with international and local market peers to identify winning strategies and monitor the developing competitive risks.
- AI risk should be reviewed to understand strategic, regulatory, ethical and technical risks. Reviews should also consider current mitigations in place, their efficiency, and recommendations to improve current practices and needed additions for newly identified risk areas.
- AI maturity must be assessed through a holistic view of the organisation, and requires active engagement across the company. This involves exploring management-backed targets and use cases, and engaging with stakeholders across technical, organisational, and partner/ecosystem domains to identify gaps, challenges and opportunities. Recommendations should focus on guidance for improving AI maturity and include high-level assessments of expected gains.
About us
Analysys Mason is a leading adviser in TMT transaction services, from deal scouting and readiness, to due diligence and post-deal value creation. We provide standalone AI risk and maturity reviews where needed, or broad portfolio scans for investors looking to understand how AI development is affecting past investments. For further information, please contact Stéphane Piot, Partner, and Christopher Ryder, Principal.
1 Klarna (2024). Klarna AI assistant handles two-thirds of customer service chats in its first month.
2 NBC New York (2024). NYC’s AI chatbot was caught telling businesses to break the law. The city isn't taking it down.
3 Ars technical (2024). Air Canada must honor refund policy invented by airline’s chatbot.
4 Analysys Mason (2023). How AI can affect the colocation market.
5 Infrastructure as a service/platform as a service.
6 NSF (2022). Annual business survey.
Author
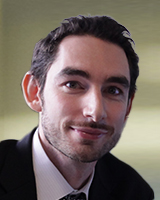