CSPs must transform their network data architecture to meet autonomous network goals
The creation of autonomous networks is a goal that many communications service providers (CSPs) aspire to achieve. For example, Orange’s CTO, Laurent Leboucher, said in a recent interview with TM Forum that the Orange Group aims to reach level 4 of the TM Forum’s model for autonomous networks 2025 for network deployment and fault monitoring.1 Automating these two processes will help Orange to improve its agility and efficiency, and its ability to address priorities including revenue growth, cost reduction and customer experience. Other CSPs, such as AIS in Thailand, China Mobile, China Telecom and MTN Group, have similar goals.
However, these objectives may be hindered by the current state of CSPs’ network data architecture, which was not designed to provide the real-time analytics that are essential for autonomous network workflows.
This article sets out three best-practice approaches that CSPs can take when seeking to modernise their network data architecture to support autonomous networks. These approaches are covered in more detail in our recent report, Modernising CSPs’ data architectures for network analytics/AI-driven automation.
CSPs’ ambitions for autonomous networks require a new operating model
CSPs that want to create autonomous networks will need a new operating model. This model will rely on a different way to implement systems that collect and analyse data (commonly known as systems of insights (SoIs)). The position and role of the SoIs will change (Figure 1). They will transition from being systems that ingest data stored within data repositories (that store data collected from systems of records (SoRs)) to being systems that connect to, and ingest, data directly from the network infrastructure. An SoI will process, analyse and share data insights with the systems of control (SoCs) to activate necessary workflows and the SoRs for real-time and offline reporting.
Figure 1: Comparing a traditional operational stack to a data-driven stack that will drive autonomous network operations
This new operating model will be necessary for cloud-native network operations.
Current network data architecture will limit CSPs’ autonomous network objectives
CSP network data architecture is crucial for enabling CSPs to define how data is collected, stored and exposed for analysis. However, existing CSP network data architecture that supports network analytics and AI use cases is not suitable to support the real-time data access and analysis requirement of the autonomous network operating model because it has the following features.
- It does not support the execution of analytics workflows and the delivery of insights in real time. Current data architecture is fed by operational support systems (OSS) SoRs, such as performance and fault management systems. These SoRs provide data offline and do not integrate directly with systems of control (SoCs), such as element and network management systems. In addition, analytical and AI use cases that are developed to consume data from SoRs often only feed insights to specific network control processes (often associated with systems from the same vendor).
- It is highly siloed and fragmented. As a result, this architecture does not provide a unified view and exposure of data for creating and operating network analytics use cases and AI models.
- It is not scalable and cost-efficient. This data architecture cannot store growing data volumes and support advanced analytics and AI functions. This is because data infrastructure runs on-premises.
CSPs that do not act swiftly to address these challenges risk not being well-positioned to address current operations and business needs. They will also be unable to pursue new opportunities presented by technologies such as generative AI (GenAI) to transform to more autonomous networks and operations.
CSPs should modernise data architecture taking into consideration three key practices
CSPs’ network data architecture should be designed to allow AI and analytics workflows to occur in real time. CSPs should adopt the following best practices modernising their data architecture.
- Data architecture should source data directly from the network. This ensures that when the network generates data, it is immediately ingested, processed and analysed. CSPs will need to develop mechanisms that parse network data directly to the data stores; eventually bypassing current generation of non-real-time SoRs to gain quicker access to, and analysis of, data.
- The data architecture should be open and de-siloed. It consolidates data from all network data sources and exposes them to any analytics/AI tool to support any network analytics use case. Such data environments provide access to a large pool of cross-domain network data that can facilitate AI model training and lifecycle management. This open data architecture will simplify data access that is needed to continuously evolve network analytics and AI use cases.
- Hybrid cloud should be adopted to scale network data architecture. With hybrid clouds, CSP data workloads can scale quickly while aligning with the regulatory requirements associated with processing network data. The data platform workloads included in the architecture can be disaggregated and executed so that sensitive and large data workloads such as data integration and transformation occur on-premises or on a private cloud network, or on public clouds with in-country or regional presence. The CSP can transfer the processed data to the public cloud for storage, management and analysis.
CSPs should expect data architecture modernisation challenges and address them to achieve their autonomous network goals
Leading CSPs have commenced their network data architecture modernisation projects. A1 Group, Orange and Vodafone have adopted a hybrid cloud model to scale and defragment network data environments. They are also developing these architectures to unify, centralise and expose data to third-party or in-house developed automation and analytics tools. An assessment of these CSPs’ implementation strategies reveals that they will encounter further challenges, including the following.
- Potential vendor lock-in. Vodafone, for example, is following a single vendor approach to transforming its network data architecture. The CSP is using proprietary data platforms offered by the public cloud providers that it engages with. This approach has lock-in implications and can have a negative impact on future data migration projects. CSPs should consider using open-source or public-cloud-agnostic data platforms, to avoid this challenge.
- Difficulty sourcing data directly from network. The maturity of existing networks makes this challenge inevitable. However, CSPs should invest in toolsets such as Apache NiFi or Kafka that can parse network data to third-party platforms to centralise data processing, management and exposure.
- Unavailability of standard data models. This is an industry-wide challenge and requires all telecoms industry stakeholders to work closely to develop and enforce the use of standard industry data models. This will be essential for ensuring that any AI or analytics tools can be served by the CSP’s network data architecture.
As CSPs modernise their network data architecture, resolving these challenges will be crucial to successfully operating autonomous networks.
Article (PDF)
DownloadAuthor
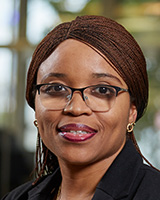