DeepSeek highlights the requirement for a new cost model to enable RAN AI to become a reality
The emergence of DeepSeek’s new AI large language model (LLM) at the end of January 2025 had a dramatic effect on US technology stocks, notably hitting the value of NVIDIA, the semiconductor maker that has made its graphical processors (GPUs) synonymous with the current AI boom. This article explores the relevance of DeepSeek’s technology for telecoms operators.
DeepSeek provides hope that an AI-native RAN could be cost-viable for mainstream operators
DeepSeek is a Chinese organisation that claims to have developed an open-source AI model that is comparable to ChatGPT, but costs only a claimed 15% of the cost to develop and requires significantly less processing power to run than the established LLMs. This would potentially reduce the requirement for NVIDIA’s advanced GPUs, hence the panic about the valuation of the chip giant, and other AI-centric vendors.
What does this mean for telecoms operators? Potentially, a great deal for those operators that are considering implementing AI in key processes. AI may be important to operators in many respects – for enhancing customer experience and engagement, improving automation and cost efficiency, and even for enabling new enterprise services. However, the biggest potential impact, and the biggest cost and risk, lies in the vision of an AI-native RAN, which has been heavily driven by NVIDIA and some advanced, mainly Asian, operators such as SoftBank and SK Telecom.
However, this is economically non-viable at the price points of current technology, so a breakthrough in the cost of implementing AI for a complex use case such as RAN is essential to enable this market to take off (whether that breakthrough comes from DeepSeek or NVIDIA or another party).
The RAN’s distributed and real-time nature makes it challenging to achieve the benefits of cloud economics
It has become almost received wisdom that the next evolution of mobile network technology will be ‘AI-native’, with AI embedded into every element of the RAN, from network management to the antenna and digital frontend. Analysys Mason’s survey of over 70 operators, conducted in October 2024, revealed that more than half of them expect to deploy some degree of RAN AI before 2030. However, many of these operators acknowledge that there is no credible economic case unless the cost of underlying processor technology is significantly reduced.
A major barrier to the large-scale implementation of cloud-based RAN has been the misalignment between the centralised nature of classic cloud economics and the distributed nature of the RAN. A RAN that has to support high levels of traffic and very quick response times requires processing power to be very close to the cell site. In a traditional network, this takes the form of a baseband unit, running on optimised, special-purpose chips, usually at the bottom of the tower. In a cloud-based RAN, the original vision was to have many sites sharing a common virtualised baseband, to maximise scalability and resource sharing. However, this came at an unacceptable cost in terms of latency for most operators, which then looked to run the most processor-intensive and time-sensitive functions (RAN Layer 1) on edge servers close to the sites.
In theory, adding AI to these servers would greatly improve the automation and intelligence of the network. But implementing high-performance servers with GPU-based RAN and AI acceleration on every 1-4 sites would come at enormous cost (a large operator such as AT&T has about 70 000 sites). One European operator confided that, according to its calculations, implementing a RAN baseband with AI embedded in Layer 1 would cost two to three times more than implementing a conventional RAN with the same traffic loads.
Although operators are interested in the potential benefits of generative AI (GenAI) in the RAN, none of them will be able to justify this kind of additional infrastructure investment. That cost would be offset somewhat by the reduced operating costs that intelligent automation promises, coupled with, to date, vaguely defined new revenue from selling spare capacity for enterprise applications and AI inferencing. Even the most-optimistic AI RAN advocate would be hard-pressed to predict an upside that would justify doubling the cost of the RAN baseband.
A resource-efficient model would help to make the AI RAN affordable and kickstart the market for all vendors
The debate about DeepSeek rumbles on, including whether it really can deliver the cost efficiencies that it promises, whether it pirated some of its technology, whether its performance matches that of ChatGPT. The answers almost do not matter; somebody is inevitably going to develop efficient LLMs that will reduce the cost of implementing GenAI at scale, because if they do not, complex and intensive applications such as the RAN will be non-viable. NVIDIA has been developing a RAN platform that includes a range of different components and price points; Qualcomm and MediaTek are pioneers in shrinking AI processing to the power and space limits of a smartphone.
The efforts of DeepSeek, Qualcomm and others will not rob NVIDIA of its market, it will expand that market and make it viable for mainstream users, rather than just the technology elite – in mobile operator terms – for all the operators that want to deploy RAN AI by 2030, rather than just for the hi-tech leaders such as SoftBank or Reliance Jio.
Operators have been cautious about the cloud so far, failing to make the edge investments that were expected a few years ago. They are reducing their capex budgets amid stagnating revenue growth, with only limited signs that they will reverse that trend. Operators such as Vodafone have spoken of very low levels of return on capex for 5G overall, and most operators insist ‘6G’ must not involve another big-bang network upgrade. Therefore, operators will need a very big confidence boost to invest en masse – either directly or via cloud partnerships – in the infrastructure required to support an AI-native RAN.
John Stankey, AT&T’s CEO, told financial analysts on the company’s 4Q 2024 earnings call on 28 January 2025: “We should expect there are going to be days we wake up like this one, when somebody comes in and says they figured out a way to get as much benefit out of the model by consuming less power or using less processing capability …. Which is going to open up and facilitate new applications and business models.”1
The operator community is waiting for that breakthrough. It may come from DeepSeek, though geopolitics may limit how far North American and most European operators can benefit from a model that is powered by Huawei processors in China; or it may come from an established player such as NVIDIA itself, or another start-up that is currently under the radar. Without such innovations, the AI-native RAN is likely to remain the preserve of a few pioneers for another generation.
1 Fortune (28 January 2025), AT&T CEO warns more DeepSeek shocks will hit U.S. tech markets.
Article (PDF)
DownloadAuthor
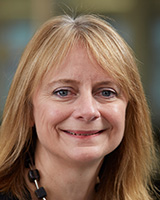
Caroline Gabriel
Partner, expert in network and cloud strategies and architectureRelated items
Article
Stakeholders must collaborate to prove the security benefits of Open RAN and de-risk early deployments
Forecast report
Mobile packet core: worldwide forecast 2024–2030
Strategy report
Considerations and strategies for Open RAN cyber security