Operators need intelligent RAN automation solutions to amplify cost savings and energy efficiency
14 February 2024 | Research
Article | PDF (3 pages) | Network Automation and Orchestration
RAN automation has been an important goal for mobile network operators (MNOs) as they accelerate the deployment of virtualised and Open RAN to support new 5G services. Intelligent RAN solutions that are integrated with AI will help operators to reduce the total cost of ownership (TCO) of the network even more so than with basic automation solutions. In addition, commitments to sustainability and environmental, social and governance (ESG) goals have made it increasingly important for MNOs to minimise energy consumption and make mobile services more sustainable. This article explores the challenges in RAN energy management and opportunities for MNOs to advance their automated Open RAN operations to reduce energy consumption and TCO.
The roll-out of 5G RAN to support high-speed mobile services is leading to rising energy consumption and operating costs
Improving energy efficiency in the RAN is one of the key priorities for many MNOs. RAN accounts for the largest share of energy costs in a mobile network – nearly 70% of an MNO’s energy consumption takes place in radio base station sites, and this cost will only continue to increase due to rising 5G traffic and demand. 5G New Radio is designed to improve energy efficiency in comparison to 4G with its ability to offer more capacity and support high volumes of good-quality data for a single base station. However, 5G uses higher-frequency spectrum bands, which results in shorter range and reduced coverage. To compensate, operators need to increase the number of cells, resulting in an overall increase in energy consumption. Furthermore, the deployment of 5G is taking place while legacy 4G networks are still in use, which has exacerbated energy efficiency and operating costs.
The NGMN Alliance was established by MNOs worldwide to work together to implement intelligence RAN energy saving features and update RAN sites with energy efficient RAN equipment and antennas. However, efficient energy management must strike a delicate balance between power savings and network quality without impeding service delivery. Efforts should be made to introduce minimal processing overhead on RAN network elements and should not affect the key performance indicators (KPIs) of the RAN service. Furthermore, conflict mitigation and triaging are imperative for maintaining stable and conflict-free network operations.
Automation will play a pivotal role in orchestrating the deployment and roll-back of energy-saving features and enable quick and automated detection of any anomalies or degradation in KPIs. This involves policy-based coordination of network configuration actions among all energy-saving features and other RAN performance-related optimisations. Validating energy savings accurately also requires sophisticated testing at scale, which necessitates the use of standard testbed configurations and methods with load generation capability.
Current RAN management systems need to be upgraded to support advanced capabilities and achieve greater automation
Many MNOs are deploying RAN optimisation and automation solutions as part of their sustainability strategies to reduce their energy consumption and operating costs. According to our recent survey of 62 Tier-1 operators, 62% of CSPs want to attain over 50% of RAN automation by 2027. CSPs are also seeking to increase their levels of RAN automation, from an average of less than 20% of processes in 2023, to a targeted average of about 70% by 2027.
The shift to Open RAN has driven ecosystem players to develop innovative solutions to improve the efficiency and scalability of next-generation RAN deployments. The adoption of the O-RAN Alliance’s service management orchestration (SMO) platform and the RAN intelligent controller (RIC) presents great potential for operators to advance their automated RAN operations, improve network performance and minimise operating costs without compromising on customer experience. Self-organising network (SON) systems have also been used as a tool for planning, configuring, managing, healing and optimising the RAN.
The RIC serves as a platform for hosting software applications that are designed to enhance management functionalities. xApps and rApps can be deployed on the RIC to enable energy management and optimisation capabilities across the radio unit (RU), distributed unit (DU), centralised unit (CU) and cloud network functions. These energy savings management applications can be coupled with a telemetry solution to retrieve and process comprehensive energy consumption data from significant points in the network enables closed-loop automation of energy use analysis, and control and facilitates the optimisation of energy consumption across the entire RAN network function lifecycle.
Investing in AI-driven automation will allow operators to minimize TCO and enable new revenues with modern RAN use cases
Many vendors are beginning to integrate AI capabilities into their intelligent RAN management and automation solutions. For example, Nokia has recently upgraded its Manta Ray SON energy portfolio to enable use cases such as AI/ML-based optimisation of base station energy-saving features. The Ericsson RAN Energy Control rApps uses AI/ML to provide an autonomous mechanism for radio power-saving features. In our survey, 35% of CSPs said that AI-enhanced power management tools were one of their two top priorities for energy efficiency.
Operators and vendors must actively work with industry groups to establish the requirements for a next-generation, cloud-based energy management platform and accelerate the adoption of AI/ML in their network automation strategies across open RAN architecture. The O-RAN Alliance has introduced several use cases in its 2023 Network Energy Savings Technical Report for network energy saving for Open and Intelligent RAN as outlined below.
- Carrier and cell switching. The control mechanism for carrier and cell switching is facilitated by the non-RT or near-RT RIC and considers overall network energy efficiency instead of local optimisation. AI/ML models can be deployed to predict expected energy efficiency enhancements, network performance and resource usage for different energy saving optimisation states.
- RF channel reconfiguration. Energy saving can be achieved by minimising the power consumption of O-RUs by switching off certain radar array antennas in a digital mMIMO architecture, allowing the number of spatial layers and single-sideband radios to be reduced. The reconfiguration depends on the management architecture model (hybrid or hierarchical) and the deployment option (non-RT or near-RT RIC).
- Advanced sleep mode selection. This involves partially switching off O-RU components to reduce power consumption. This requires the O-DU and O-CU to understand the number, depth and other sleep mode capabilities of the O-RU to drive sleep mode policies. For optimisation, O-RU and O-DU need to report energy efficiency related measurements and KPIs from which the power saving can be observed.
- O-Cloud resource energy saving mode. Reduce power consumption of O-Cloud components through non-RT RIC guided actions without compromising on network performance. This includes adaptive shutdown of hardware, dynamic scaling of network function workloads and CPU power optimisation. This category of use cases is control and configured by the non-real-time RIC.
Article (PDF)
DownloadAuthor
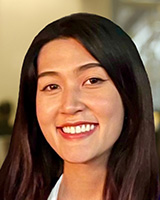
Michelle Lam
AnalystRelated items
Article
CSPs to spend USD13.8 billion on network automation and orchestration systems and services in 2029
Article
Vendors must adopt GenAI solutions quickly to maintain a competitive edge in the telecoms market
Article
Vendors need a new approach to product pricing for the next generation of telecoms operational applications